Navigating the Pharmaceutical Industry's Data Challenges
Data-driven decisions are paramount in the pharmaceutical industry. Yet, we continue to face challenges around data use in the world of pharma.
During a recent discussion, I had the opportunity to share my thoughts on some of the most pressing data-related issues facing the industry today, as well as potential solutions. Here’s a look.
The Trust Conundrum in the Age of AI
One of the most significant hurdles we face in the pharmaceutical industry today is trust. Trust has always been essential in this industry, but in today’s era of artificial intelligence (AI), it takes on an entirely new dimension.
We not only need to establish trust in our institutions and organizations but also cultivate confidence in machine intelligence. AI is playing an increasingly significant role in drug discovery, treatment optimization, and patient care.
To gain acceptance, we must instill trust in the algorithms and systems underpinning these critical processes, and as the pharmaceutical ecosystem evolves – even as regulations continue to tighten – trust becomes an even more central issue.
The Effect on Consumer Confidence
The paradox we confront is that while we have more data at our disposal than ever before and analytical tools have reached new levels of sophistication, trust in the industry appears to be on the decline.
In fact, only about one-third of customers believe that companies are currently using their data responsibly (McKinsey), and 81% of people have privacy concerns over how AI is used for recommendations, customer service and support (CDP.com).
On the other hand, a reported 80% of pharmaceutical and life sciences professionals are currently using AI for drug discovery, and it’s paying off: AI applications can potentially create between $350 billion and $410 billion in annual value for pharmaceutical companies by 2025 (zipdo.co).
So how can we increase consumer confidence while leveraging the full potential of these new technologies?
To tackle this issue head on, we must embrace change management and adapt our data governance frameworks.
Our primary objective should be to enhance transparency and trust among all stakeholders, including pharmaceutical companies, payers, healthcare providers, and, perhaps most crucially, patients.
Learning from Successes and Failures
During our discussion, I had the pleasure of sharing some specific use cases and examples that garnered significant engagement & approval from the audience.
Patient Engagement Data: The transformative potential of gathering data from the patient engagement journey cannot be overstated. This data can revolutionize how pharmaceutical companies approach healthcare providers (HCPs) and patients alike. By merging patient experience data with operational data, we can optimize our engagement strategies. As this data continues to expand, so too do the opportunities for more effective engagement.
Contact Center Augmentation: Augmenting contact center interactions can lead to more meaningful engagement while also partially replacing some functions typically handled by contact center operators. The key lesson here is the importance of democratizing data capabilities and ensuring that decision-making power is accessible to both data analytics teams and business users. This approach will set us apart in the evolving landscape of the life science industry.
Balancing Global and Local Analytics Capabilities
Another critical point I addressed was how to strike the right balance between global and local advanced analytic capabilities in the pharma sector. Here are some things that can help:
Data Democratization: Our approach has always been to prioritize goals. In the age of generative AI, I advocate for a data democratization model that spans the entire organization. This approach entails working closely with business users to extend data capabilities across the organization. With modern generative AI tools becoming more accessible, involving stakeholders from all sides of the business in driving outcomes is essential.
The Evolving Role of Data Teams: With Large Language Models (LLMs) gaining prominence, data governance is taking on an even more critical role. As more individuals within the organization experiment with data, data teams may find themselves evolving into facilitators, ensuring the responsible and ethical use of data in alignment with our business objectives.
Final Thoughts
The pharmaceutical industry faces several challenges in the realm of analytics and data management, with trust being a paramount concern.
Addressing these challenges involves embracing change, democratizing data capabilities, and evolving the role of data teams, but if we can learn from both successes and failures and while fostering a culture of data-driven decision-making, the pharmaceutical sector can confidently navigate the shifting landscape.
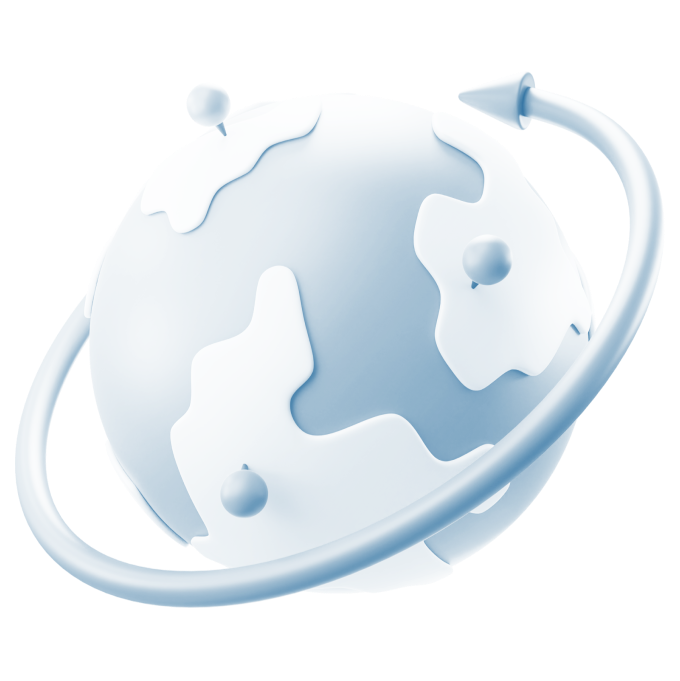
Need help leveraging AI effectively?
We can help. Connect with Customertimes to find AI solutions that increase your analytics and data management capabilities, regardless of industry.
Let's connect-fm-converted.jpg)
Konstantin Perederiy
With more than 15 years of IT industry experience, Konstantin Perederiy is passionate about creating opportunities for businesses to maximize their investment in the digital economy. As a sales leader at a global organization, he helps teams across every industry design and implement innovative digital products and solutions using cutting-edge AI, CRM, and ERP business applications.
-fm-converted.png)
Overcoming Apprehension around Emerging Technologies
-fm-converted.png)
How to navigate the challenges of implementing innovative tech in healthcare
-fm-converted.png)
How AI is Improving the Work of Pharma Sales Reps
-fm-converted.png)
Insurance Fraud: Facing Up to the $300 Billion Problem
-fm-converted.png)
Keeping Up With Consumer Expectations in the Financial Services and Insurance In...
-fm-converted.png)
Navigating Data Engineering Challenges in the Insurance Industry
-fm-converted.png)
AI's not so nice side: Key concerns businesses are thinking about
-fm-converted.png)
What’s the difference between Monolithic and Microservices architecture?
-fm-converted.png)
Artificial Intelligence: Is it a Force for Good in Business?
-fm-converted.png)